What is UAVid?
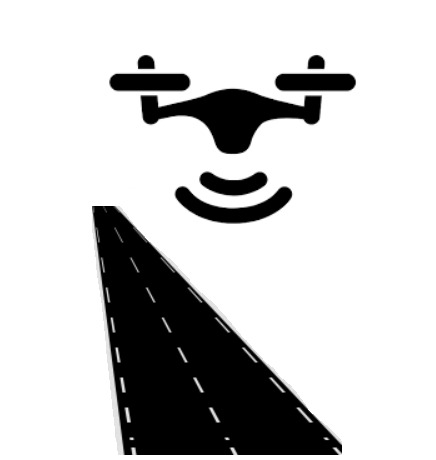
The UAVid dataset is an UAV video dataset for semantic segmentation task focusing on urban scenes. It has several features:
- Semantic segmentation
- 4K resolution UAV videos
- 8 object categories
- Street scene context
High resolution quality
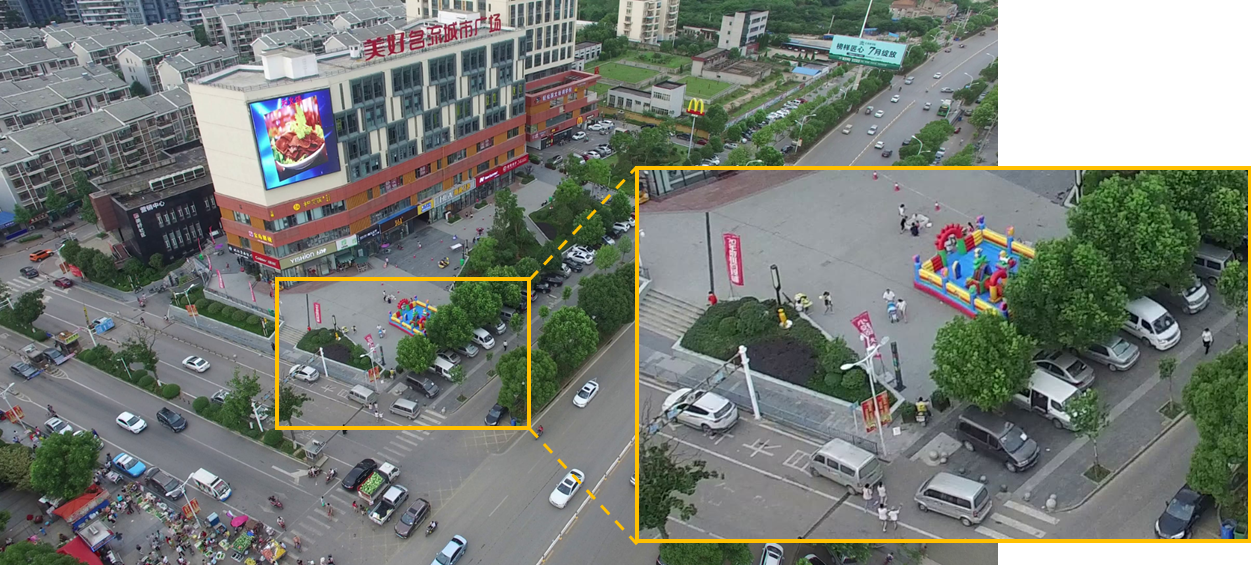
The images are captured in very high resolution with detailed scenes.
What are the categories?
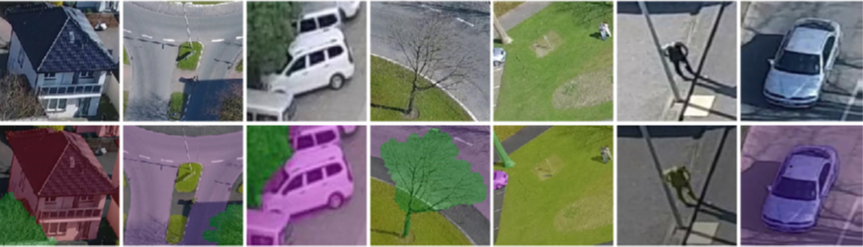
There are 8 categories in total:
- Building
- Road
- Static car
- Tree
- Low vegetation
- Human
- Moving car
- Background clutter
News
- UAVid 2020 test set ground truth is publicly available! Download available on EOStore (password: uavid2023) UAVid-depth dataset is online! Task: Self-supervised monocular depth estimation from UAV videos. Dataset description and download available on DANS UAVid 2020 version is online! Dataset download is available now! UAVid 2020 version has 42 sequences in total (20 train, 7 valid and 15 test). Besides the original 30 sequences (UAVid10 version), another 12 sequences have been collected to further strenghthern the dataset.
- Evaluation server is online. Both of the UAVid10 and the UAVid2020 can be evaluated on the Codalab. Experiments on UAVid2020 are recommended. Go to benchmark page for more details.
Copyright
UAVid dataset and UAVid-depth dataset are copyright by us and published under the Creative Commons Attribution-NonCommercial-ShareAlike 4.0 License. This means that you must attribute the work in the manner specified by the authors, you may not use this work for commercial purposes and if you alter, transform, or build upon this work, you may distribute the resulting work only under the same license.
Citation
Please cite our paper if you find our UAVid dataset useful. Bibtex references are as follows,
@article{LYU2020108, author = "Ye Lyu and George Vosselman and Gui-Song Xia and Alper Yilmaz and Michael Ying Yang", title = "UAVid: A semantic segmentation dataset for UAV imagery", journal = "ISPRS Journal of Photogrammetry and Remote Sensing", volume = "165", pages = "108 - 119", year = "2020", issn = "0924-2716", doi = "https://doi.org/10.1016/j.isprsjprs.2020.05.009", url = "http://www.sciencedirect.com/science/article/pii/S0924271620301295", }
When using the UAVid-depth dataset in your research, please cite: @article{uaviddepth21, Author = {Logambal Madhuanand and Francesco Nex and Michael Ying Yang}, Title = {Self-supervised monocular depth estimation from oblique UAV videos}, journal = {ISPRS Journal of Photogrammetry and Remote Sensing}, year = {2021}, volume = {176}, pages = {1-14}, }
Privacy and Cookies
Organization
- Semantic Labelling with Video Support
- Semantic Labelling with Images Only
- UAVid Toolkit (python)
The UAVid dataset provides images and labels for the training and validation set, and images only for the testing set. All sequences are provided with the corresponding videos. Image and label files are named according to the 0-based index in the video sequence.
If you only need images and labels from the UAVid dataset without video support, please use the following link.
UAVidToolKit provides basic tools for easier usage of the UAVid dataset. Including label conversion, label visualization, performance evaluation
- Semantic Labelling
- Evaluation Metric
The task for UAVid dataset is to predict per-pixel semantic labelling for the UAV video sequences. The original video file for each sequence is provided together with the labelled images. Currently, UAVid only supports image level semantic labelling without instance level consideration.
The semantic labelling performance is assessed based on the standard Jaccard Index, more known as the PASCAL VOC intersection-over-union metric.
TP, FP and FN are the numbers of true positive, false positive and false negative respectively,
which can be calculated through the confusion matrix determined over all data from test split.
The goal for this task is to achieve as high IoU score as possible.
For UAVid dataset, clutter class has a relatively large pixel number ratio and consists of meaningful objects,
which is taken as one class for both training and evaluation rather than being ignored.
- Evaluation
The ground truth of test set is made publicly available in 2023.
- Annotation Method
- Pixel annotation: label pixels in basic scribbling style.
- Super-pixel annotation: automatically partition the image into super-pixels first, and label super-pixels instead.
- Polygon annotation: draw polygons intead of pixels. Pixels in the polygon are labelled the same class.
- Video Labelling Tool
All the labels are acquired with our home-made video labeller tool. Three annotation methods are provided:
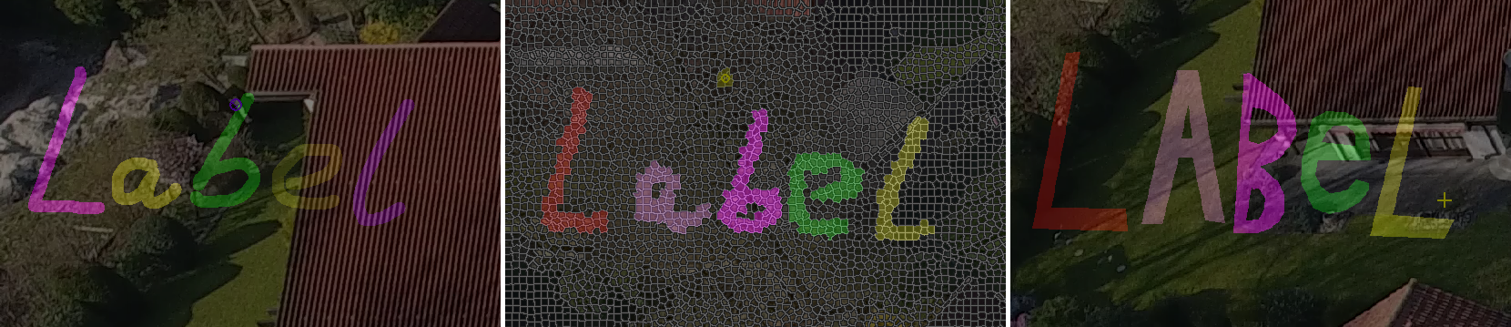
This tool is used for making video semantic labeling ground truth data. It has been used for UAVid dataset.
[Video Semantic Labeler Link]